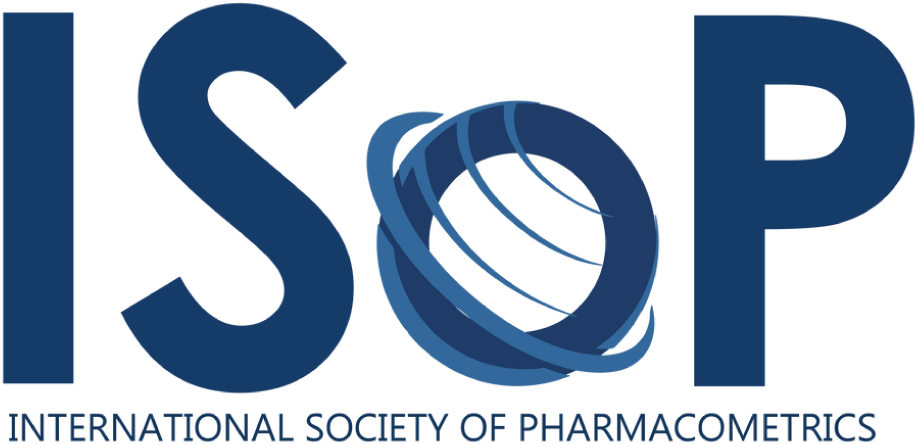
Population joint modeling of SARS-CoV-2 neutralizing titer dynamics after COVID-19 mRNA vaccine booster administration
-
You must log in to register
- Non-member - Free!
- Member - Free!
- Guest User - Free!
Authors
Jiawei Zhou, PhD - Pfizer, Inc./ Rohit Rao, NA - Senior Principle Quantitative Systems Pharmacologist, Pharmacometrics and Systems Pharmacology, Pfizer Inc.; Xia Xu, NA - Executive director, Vaccine Research and Development, Pfizer Inc.; Jim Hughes, NA - Pharmacometrician, Associate Director, Pharmacometrics and Systems Pharmacology, Pfizer Inc.; Kayvon Modjarrad, NA - Executive director, Vaccine Research and Development, Pfizer Inc.; Cody Herron, NA - Senior Quantitative Systems Pharmacologist, Pharmacometrics and Systems Pharmacology, Pfizer Inc.; Richard Allen, NA - QSP Group Lead, Pharmacometrics and Systems Pharmacology, Pfizer Inc.; Patanjali Ravva, NA - Pharmacometrics Group Lead, Pharmacometrics and Systems Pharmacology, Pfizer Inc.; Juleen Gayed, NA - Director, Vaccine Research and Development, Pfizer Inc.; Nicholas Kitchin, NA - VP, Vaccine Research and Development, Pfizer Inc.; Kena Swanson, NA - VP, Vaccine Research and Development, Pfizer Inc.; Cynthia Musante, NA - VP Scientific Research, Pharmacometrics and Systems Pharmacology, Pfizer Inc.; Timothy Nicholas, NA - Head of Technology & Innovation, Pharmacometrics and Systems Pharmacology, Pfizer Inc.
Abstract
Objectives: The global COVID-19 pandemic prompted the development of the BNT162b2 mRNA vaccine. This analysis aims to assess if COVID-19 mRNA vaccines adapted for different SARS-CoV-2 variants (original BNT162b2 monovalent vs Omicron BA.1 bivalent) impact the half-life of vaccine-induced SARS-CoV-2 strain-specific neutralizing titers. The influence of other intrinsic and extrinsic factors on neutralizing titer half-life were also investigated.
Methods: A model was developed using longitudinal SARS-CoV-2 50% geometric mean neutralizing titer (NT50) data from subjects in a phase 3 trial [1], who received a fourth dose of either 30 ug of the original monovalent BNT162b2 vaccine or the OMI BA.1 bivalent vaccine (15 ug original wild-type + 15 ug Omicron BA.1). Neutralizing antibody titers against three SARS-CoV-2 strains (wild-type, Omicron BA.1 and BA.4/5) were assessed for all participants. To enable direct comparisons, a joint population pharmacometrics model that simultaneously incorporated data from all three strains was developed. Three covariate selection methods were used to identify factors influencing the joint model: (a) stepwise covariate selection with expert pharmacometrician (PMx) input; (b) the Perl-Speaks-NONMEM Stepwise Covariate Model (PsN-SCM) tool; and (c) a machine learning approach [2]. External validation using data from an ongoing phase 3 clinical trial was conducted, comparing the generalizability of the covariate models selected by the different methods. The most effective model was used to estimate the NT50 half-life and compared these results between the two vaccine types.
Results: Among the three covariate selection methods, the machine learning approach showcased exceptional computational efficiency and rapid selection. However, the PMx-selected model outperformed in both internal and external validation. The PMx-selected final model revealed that covariates like time since the first COVID-19 vaccination, and the evidence of prior SARS-CoV-2 infection at baseline were associated with the dynamics of NT50 titers. The bivalent vaccine was found to induce comparable terminal half-life across all three strains compared to the original monovalent booster vaccine. Subjects with prior SARS-CoV-2 infection at baseline exhibited longer half-life compared to those without evidence of prior infection.
Conclusions: Our study provides a joint model to estimate COVID-19 mRNA booster vaccine half-life. While machine learning was most efficient for covariate selection, the PMx expert-selected model performed better.
Citations: [1] Winokur, Patricia, et al. "Bivalent Omicron BA. 1-Adapted BNT162b2 booster in adults older than 55 years." New England Journal of Medicine 388.3 (2023): 214-227.
[2] Janssen, Alexander, et al. "Application of SHAP values for inferring the optimal functional form of covariates in pharmacokinetic modeling." CPT: Pharmacometrics & Systems Pharmacology 11.8 (2022): 1100-1110.
Keywords
Joint pharmacometrics model, COVID booster vaccine, machine learning
Date of Conference
November 10-13, 2024
Conference Location
Phoenix, Arizona, USA
DOI
10.70534/CLMY5516
Key:




