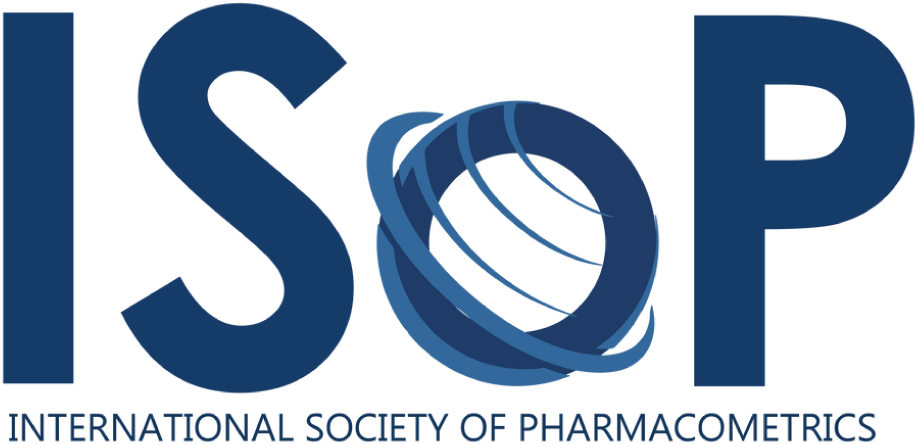
Estimation and variable selection in high dimensional setting in nonlinear mixed effects models
Recorded On: 03/31/2025
-
You must log in to register
- Non-member - Free!
- Member - Free!
- Guest User - Free!
We consider nonlinear mixed effects models including high-dimensional covariates to model individual parameters variability. The objective is to identify relevant covariates and estimate model parameters. We combine a penalized LASSO-type estimator with an eBIC model choice criterion to select the covariates of interest. Then we estimate the parameters by maximum likelihood in the reduced model. We calculate the LASSO-type penalized estimator by a weighted proximal gradient descent algorithm with an Adagrad-type adaptive step. This choice allows us in particular to consider models that do not necessarily belong to the curved exponential family. We illustrate the performance of the method in a nonlinear mixed-effects logistic growth model.
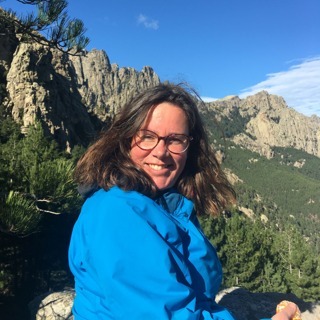
Estelle Kuhn
Researcher in Statistics
INRAE - University Paris Saclay
Estelle Kuhn, Senior Researcher in Statistics, at INRAE-University Paris Saclay I am senior researcher in statistics at INRAE-University Paris Saclay in the Dynenvie team at the Applied Mathematics and Computer Science from Genome to Environment lab (MaIAGE). My current research topics concern inference and variable selection in high-dimension setting in latent variable models such as mixed and joint models. I am also interested in hybrid models combining mechanistic and statistical models. I develop stochastic optimization algorithms such as expectation maximization and gradient descent adapted to different contexts. I apply these methodologies in strong collaboration with colleagues at interfaces, particularly in plant breeding and animal genetics.